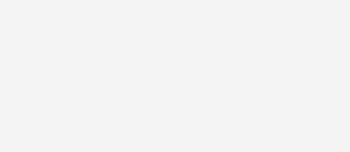
How machine learning can support and guide ESG reporting
If we acknowledge that data is a vital component of establishing a successful ML solution, then the need to understand what data a company holds, its quality and the relevant expertise required to transform and apply that data in an ML environment becomes clear. While these factors are important for any successful ML solution, they are vital when considering ML models that support and guide ESG reporting.
Strengthen trust
When applied to ESG reporting, ML models are created using potentially sensitive information, particularly when analysing social data. While data held by human resources (HR) can be a rich source of information, companies must have the parameters and guards in place to ensure that data privacy laws are not being breached. In practice, companies need to develop a high degree of employee trust underpinned by ethically sound business practices to use such information.
Be aware of data bias
Awareness of any bias in company data is essential for ESG reporting accuracy. Care is needed on data related to gender, age, or part-time and overseas workers to ensure groups are represented correctly and fairly so that the ML algorithms can predict data trends. It’s about getting the balance right between including and excluding data points to ensure that the ML model is not guided in a particular way that reduces the accuracy of information that informs reporting. For example, using techniques like oversampling to increase underrepresented classes or undersampling that reduces overrepresented classes. Also, by generating synthetic data to balance the representation of different groups.
Consider data complexities
Data used to inform ML models can be gathered from various internal and external sources for ESG reporting purposes. Internal sources include enterprise resource planning (ERP), client relations, inventory and asset management systems. Externally, several publicly available sources can be used to scrape data where there is a lack of internal data. However, data for non-financial reporting can be inconsistent and complex. For example, data used to measure a building's carbon footprint depends not only on the materials used and energy efficiency, but also on the associated supply chain. This makes accurate training of an ML model more challenging. It is therefore important for companies to understand the data points included and the parameters learned by a model to determine its predictive accuracy and overall reliability in supporting business decisions.
Combine technologies
While ML models support the data analysis process, using AI to generate reports from data and explain correlations offers additional benefits. Combining discriminative and generative AI enables organisations to perform advanced analytics on their own data, make accurate predictions about key metrics, and automatically process them into a report. However, companies must be aware that people can often attribute too much authority and trust to machine-derived information. It’s important, therefore, to ensure human oversight is in place so that reports are reliable.
ML can undoubtedly support and guide ESG reporting. However, companies need a clear understanding of the challenges alongside the benefits. ML models are designed to provide an answer. Data quality, expertise, and strong oversight measures are paramount to ensure those answers are accurate and trustworthy.
Want to know more?
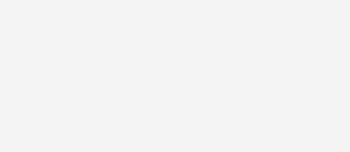